Pattern recognition is a crucial aspect of machine learning. Its primary goal is to identify and categorise patterns in data, making it an essential technique in various domains.
In the past, pattern recognition relied on manual efforts to spot regularities in data. Yet advancements in machine learning have revolutionised this process by automating it and enhancing its efficiency significantly.
Nowadays, machine learning algorithms analyse vast datasets, learn from the information contained within them, and accurately recognise recurring patterns. The financial sector extensively utilises pattern recognition for several purposes.
Here, we take a closer look at the pattern recognition meaning, how it works and key use cases in finance.
What are Patterns in Data?
Patterns are recurring sequences or regularities found within data. They represent an order or predictable organisation of data.
In the realm of computational thinking, patterns go beyond mere repetitions; they embody relationships, trends, and structures within the data that offer valuable insights upon analysis.
For instance, in open banking, recognising patterns in customer transaction data can unveil spending behaviours, enabling personalised financial products and services. Understanding and leveraging these patterns is crucial for unlocking the full potential of data, establishing pattern recognition as a cornerstone in modern financial technology solutions.
How Does Pattern Recognition Work?
In machine learning, pattern recognition follows a systematic approach to extract valuable insights from data.
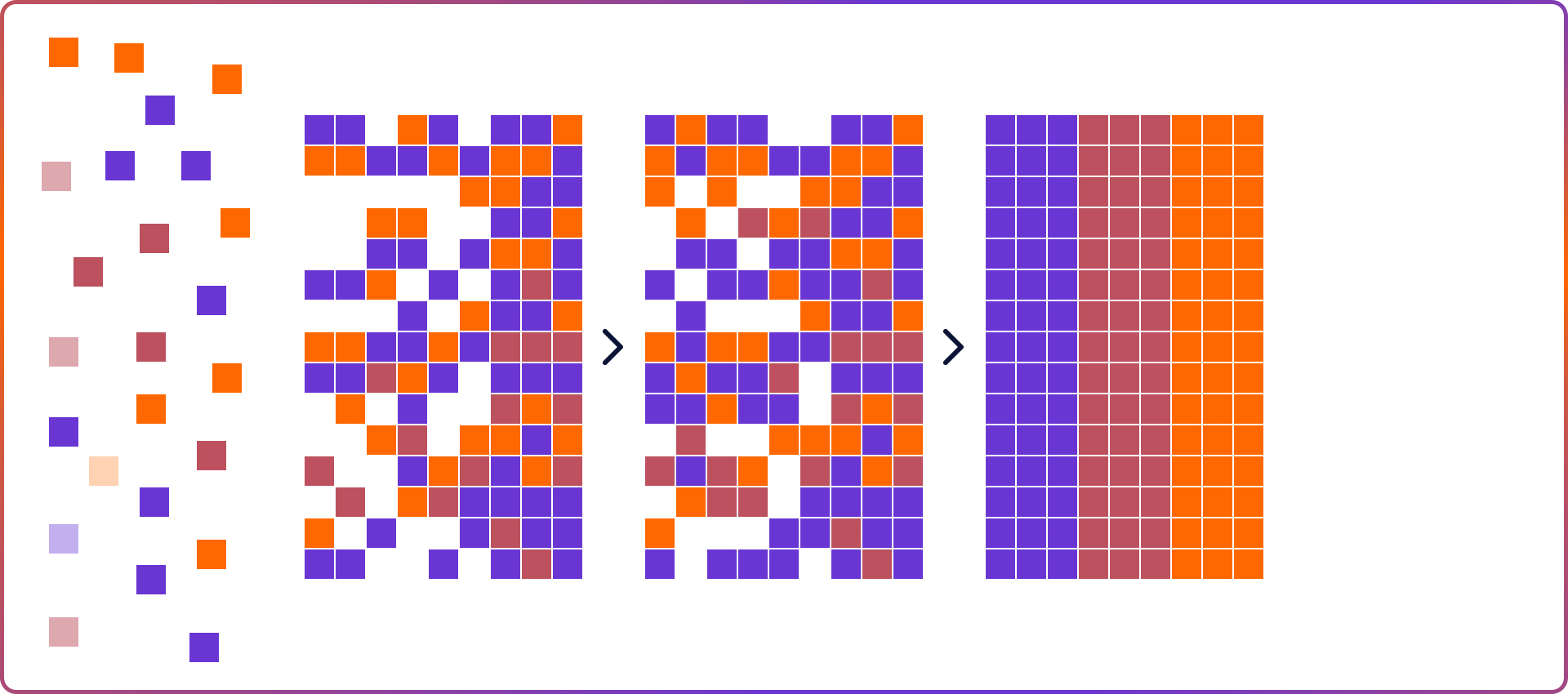
1. Acquiring & Preprocessing
The first step involves acquiring relevant data from various sources. Next, the data undergoes preprocessing to remove inconsistencies and inaccuracies, ensuring accurate results.
2. Representation
After preprocessing the data, the next step is data representation. This involves transforming the preprocessed data into a format that machine learning algorithms can easily analyse. One common approach is to convert the data into vectors, graphs, or other structures that capture the important characteristics of the data.
3. Decision making
In this stage, machine learning algorithms thoroughly analyse the data representation to identify and categorise patterns effectively. These algorithms continuously learn from the data, progressively enhancing their accuracy and efficiency over time.
The valuable insights gathered from this stage are then utilised to make well-informed decisions in various domains like fraud detection, market analysis, and other applications.
Pattern Recognition Use Cases in Fintech & Finance
Pattern recognition plays a crucial role for both fintechs and financial institutions as they harness data to make better decisions. One vital application entails detecting fraud, where algorithms analyse transaction data to identify unusual patterns that hint at fraudulent activity. By doing so, these algorithms effectively mitigate the risks.
Pattern recognition excels in the domain of financial data analysis. By examining extensive datasets, it uncovers valuable insights into market trends, customer behaviours, and investment opportunities. These findings empower financial institutions to personalise their services, optimise strategies, and maintain a competitive edge.
Through pattern recognition, both fintechs and established financial organisations can enhance operational efficiency while providing clients with more personalised and secure products.